Top trends in data science for 2022
Published
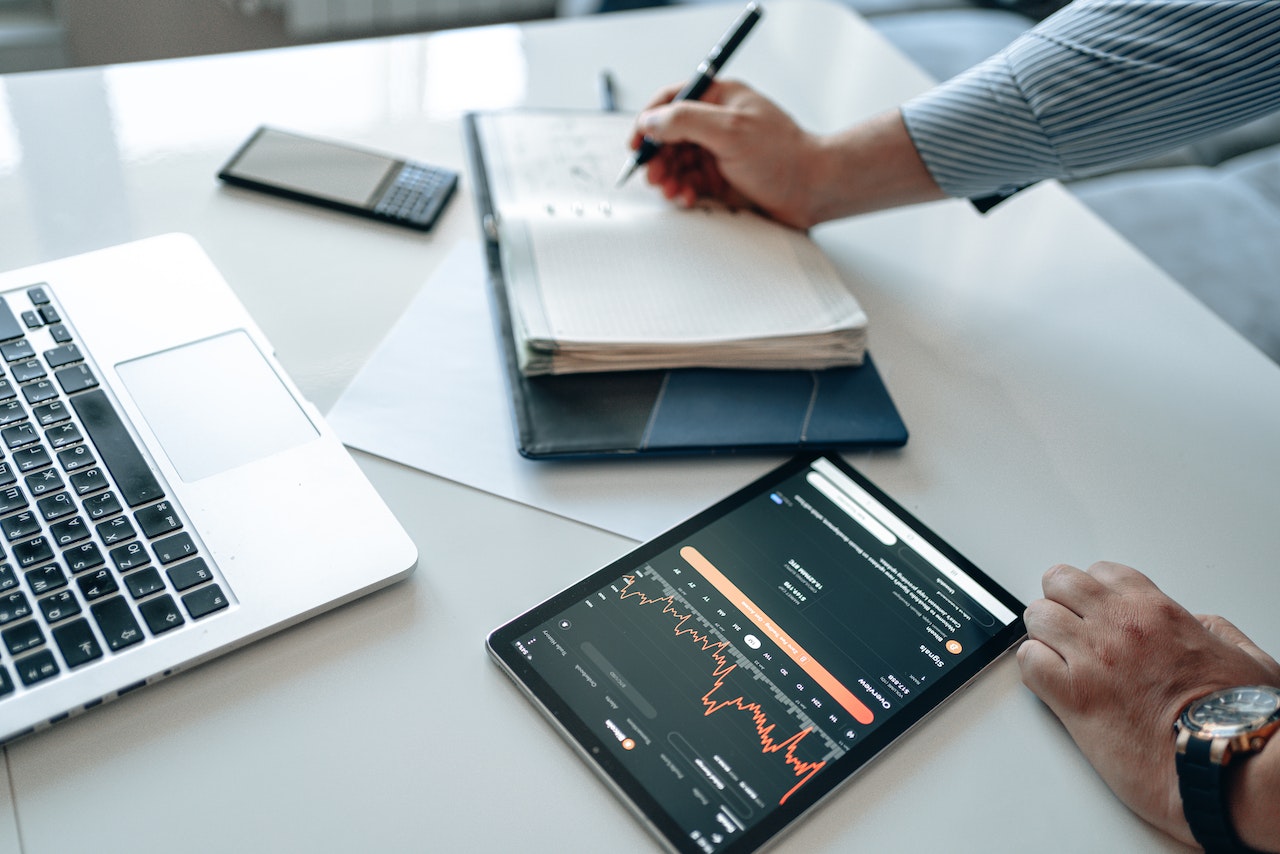
Big data is no longer a new concept for companies. They have become an integral part of the business wheel, especially for companies that swear by how this data can be used to generate insights. Data science is where science meets AI. Despite the pandemic, this area has only grown. The report " State of Data Science 2021 " by Anaconda states that only 37% of companies are investing in Data Science have reduced.
If you want to acquire new data science skills or expand your existing skills, Skillshare is for you. Please click here to access Skillshare's learning platform and gain new insights into a wide variety of topics.
Data science is one of the fastest growing areas in the technology industry. It is also an area that is changing the way we approach data and analytics both in the workplace and in our daily lives. Whether you consider yourself an expert or a complete newbie, these 10 trends in data science will impact your business in the future.
1. Cloud migration boom
68% of CIOs cited public cloud migration or private cloud expansion as the top driver of IT spending in 2020. Enterprises will soon begin preparing for application migration by upgrading their on-premises applications containerize. Reasons for this include cost considerations, chip shortages and the need for scalability. Enterprises will migrate their online transaction processing systems, data warehouses, web applications, analytics and ETL to the cloud.
Companies that already have hybrid or multi-cloud deployments will focus on porting their data processing and analytics. In this way, they will be able to switch from one cloud service provider to another without having to worry about lock-in periods or the use of specific point solutions.
2. Growth of predictive analytics
By analyzing data from more than 100 million subscribers, Netflix was able to influence more than 80% of the content viewed by its users, thanks to precise data insights.
Predictive analysis involves predicting future trends and forecasts using statistical tools and techniques that leverage past and existing data. Using predictive analytics, companies can make insightful business decisions that help them grow. Thanks to data-driven insights gained using predictive analytics, they can rethink their strategy and revise their goals.
The global predictive analytics market is expected to grow to $21.5 billion by 2025, with a CAGR of 24.5%. The incredible growth predicted here is due to the adoption of digital transformation across a number of companies. Microsoft CEO Satya Nadella is quoted as saying, "We've seen two years of digital transformation in two months."
3. AutoML
Automated Machine Learning (AutoML) is one of the latest trends driving the democratization of data science. A large part of a data scientist's job is data cleaning and preparation, and each of these tasks is repetitive and time-consuming. AutoML ensures that these tasks are automated and includes the creation of models, algorithms and neural networks.
AutoML is essentially the process of applying ML models to real-world problems by leveraging automation. AutoML frameworks help data scientists with data visualization, model understandability, and model deployment. The main innovation is hyperparameter search, which is used for preprocessing components, selecting the model type and optimizing their hyperparameters.
4. TinyML
TinyML is a type of ML that shrinks deep learning networks to fit on any hardware. Its versatility, tiny form factor, and cost-effectiveness make it one of the most exciting trends in the field of data science that can be used to develop a range of applications. It embeds AI into small pieces of hardware and solves the problem of embedded AI, namely energy and space.
Machine learning on devices is used in a variety of areas. From building automation to drug development and testing, it enables fast iteration cycles, more feedback and the opportunity to experiment further. Pattern recognition, audio analysis and voice-controlled human-machine interfaces are the areas where TinyML is used extensively.
Audio analytics helps with child and elderly care, device monitoring, and security. In addition to audio analysis, TinyML can also be used to recognize images, movements and gestures. According to McKinsey, there are currently more than 250 billion embedded devices worldwide. TinyML can bridge the gap between edge hardware and device intelligence. With the advent of newer human-machine interfaces, TinyML can embed AI and data processing in a cheaper, scalable and more predictable way. The number of TinyML devices shipped is expected to increase from 15 million in 2020 to 2.5 billion in 2030.
5. Cloud-native solutions are becoming a must-have
The term “cloud native” is generally used to describe container-based environments. They are used to develop applications built with services packaged in containers. The containers are deployed as microservices and managed via agile DevOps processes and continuous delivery flows on an elastic infrastructure. A cloud-native infrastructure includes software and hardware used to run the applications effectively. Infrastructure also includes operating systems, data centers, delivery pipelines, and a range of apps that support them.
Thanks to the widespread adoption of digital transformation, most companies now operate in a cloud-based environment. Building an on-premise infrastructure is associated with high costs - one more reason why companies today rely on a cloud-based environment. This also includes the introduction of cloud-native analytics solutions that create detailed analyzes in the cloud.
6. Advanced consumer interfaces
In the near future, there may be an AI agent in the form of an interface to help you shop. You could purchase your products in VR, get a taste of the product via audio, or through an advanced consumer interface. Advanced consumer interfaces can take various forms, such as: B. AR on the cell phone or a communication interface such as a brain-computer interface (BCI). These technologies are having a real impact on the way we shop. Even your Zoom meetings could be replaced with new advanced user interfaces. The metaverse that Facebook, Microsoft and other companies are creating will be part of this expanded consumer interface.
The technologies that will give a boost to advanced consumer interfaces are IoT, VR, AR, BCI, AI speakers, AI agents, and so on. All these technologies will evolve into a new paradigm in which artificial intelligence will act as an intermediary.
7. Better data regulation
According to G2, 2,000,000,000,000,000,000 bytes of data are created every day across all industries. That's 18 zeros. Does this draw your attention to the importance of data regulation? It really should.
Optimizing big data shouldn’t be an afterthought. With data driving every aspect of AI, predictive analytics, etc., companies must handle their data carefully. Data protection is no longer a buzzword. According to a report from the 2019 Cisco Consumer Privacy Survey, 97% of companies recognize that they see benefits such as competitive advantage and investor attractiveness when they invest in data protection.
With the advent of AI in industries such as healthcare, sensitive EMR and patient data cannot be compromised. Data Privacy by Design will help create a more secure approach to collecting and processing user data while the machine learns to do it itself.
What we do, how we move and build in the cloud should also be examined from a political regulation perspective. The speed at which data science and its technologies are evolving is immense. There are hardly any efforts to regulate data protection or to ensure the security and inviolability of customer data. AI systems could lead to a massive crash if there is no regulator to ensure their maintenance.
8. AI as a Service (AIaaS)
This refers to companies that offer out-of-the-box AI solutions that enable customers to implement and scale AI techniques at low cost. Recently, OpenAI announced that it would make its Transformer language model GPT-3 available to the public as an API. AIaaS is one of the latest trends in which state-of-the-art models are offered as services.
The future of this technology will be characterized by clearly defined and self-contained functions. For example, a manufacturing company will use one service to create a chatbot for internal conversation and another service for inventory forecasting. Thanks to the increasing number of AI models with expertise, complex algorithms that provide specific solutions can be built on demand.
One of the biggest challenges associated with AIaaS is meeting compliance requirements. If you are a business that can meet your compliance and regulatory obligations, then AIaaS is a great way to develop AI solutions quickly and at scale.
The AIaaS market is expected to reach $43.298 billion by 2026, with an incredible CAGR rate of 48.9% during 2021-2026. AIaaS looks extremely promising for 2022 and beyond , and we will likely see a number of companies leveraging AI using this technology.
9. Complexity of training data
Although there is always talk about how data is the new oil and how important it is for businesses, most of this collected data remains unused. It is also known as “dark data” and is usually only collected, processed and stored for compliance purposes. Additionally, 80-90% of the data companies generate today is unstructured, making it even more difficult to analyze.
Building credible machine learning models requires large amounts of training data. Unfortunately, this is one of the main reasons hindering the application of supervised or unsupervised learning. There are certain areas where large data sets are not available, which Data science activities can seriously hinder.
Transfer learning, generative adversarial network (GAN), and reinforcement learning solve this problem by reducing the amount of training data required or generating enough data to teach models.
For a machine to learn what you want to teach it requires at least hundreds of thousands of examples. Transfer learning ensures that this number is reduced to a few hundred. GANs are great for creating data that reinforcement learners can interact with in a highly simulated environment. GAN is the technology behind deep fake that creates lifelike images and videos.
10. Human jobs will remain safe
People assumed that AI would take over their jobs. Nothing could be further from the truth. AI has helped streamline human work more than ever. Even though the tools provided by AI get things done faster and are less prone to errors, your tasks aren't going away any time soon.
Companies that use artificial intelligence for data analysis are able to achieve great success through data-driven business decisions. The best thing about AI is that it sifts through massive amounts of data, finds patterns, analyzes them, and converts them into insightful information.
Although people will be replaced in some areas, there will be no shortage of jobs and no one needs to panic. The human factor will always play an important role, there is no doubt about that. Data science is not yet so advanced that its AI can replace the human mind. Data scientists will interpret the data using AI algorithms, helping companies scale their operations faster and more efficiently.
conclusion
Data science encompasses both practical and theoretical applications of ideas and leverages technologies such as big data, predictive analytics, and artificial intelligence. In this article, we have discussed 10 of the top trends in data science for 2022 and beyond. It is expected that the market for Big Data and data analytics will reach more than $421 billion by 2027. The field of data science is growing at a tremendous pace, and companies are putting their hearts into it to ensure they don't fall behind.