The role of data engineering in modern business intelligence
Published
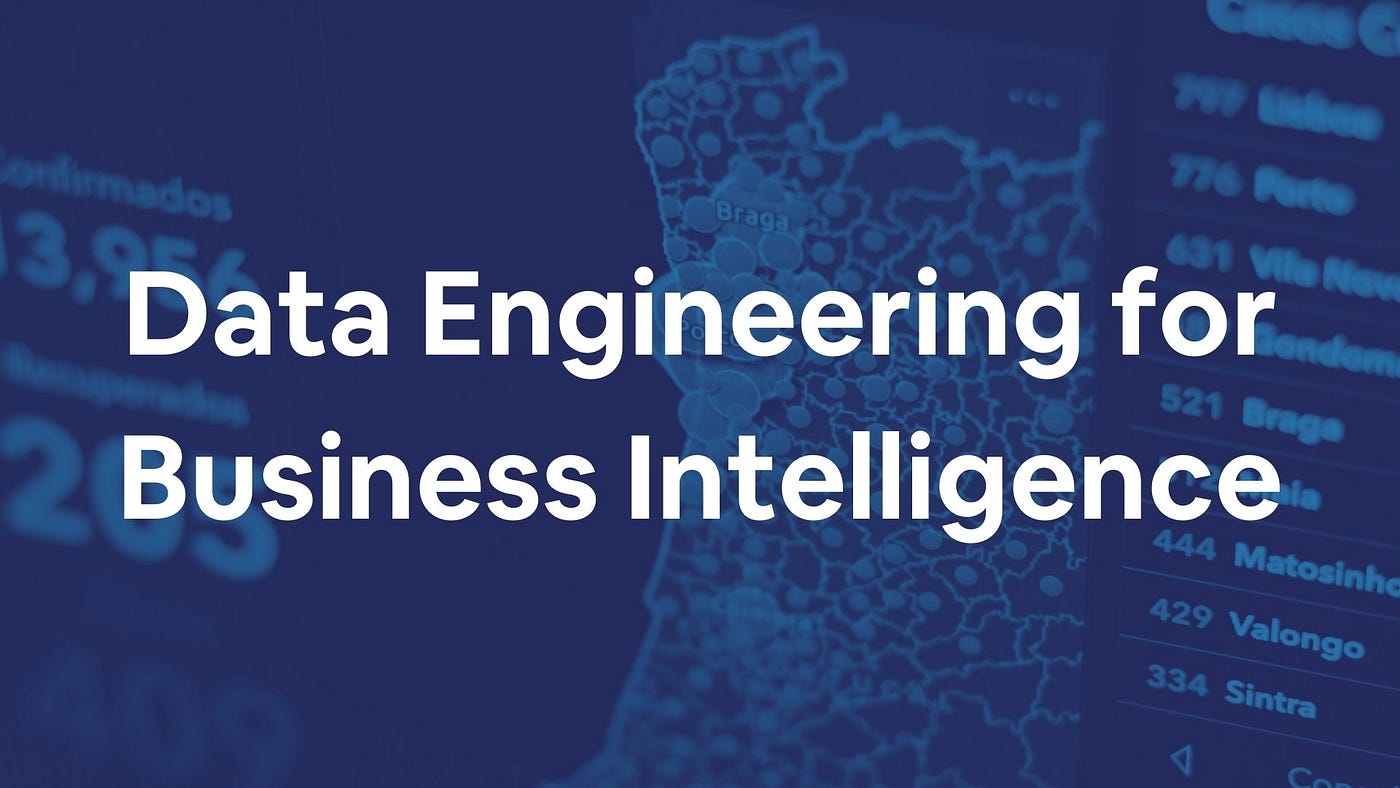
Why data engineering is crucial for modern business intelligence
In today's data-driven business landscape, companies must be able to quickly and accurately derive insights from large amounts of data to remain competitive. This is where data engineering comes into play. Data engineering is the process of developing, building, and maintaining the infrastructure that supports the collection, storage, processing, and analysis of data.
Without appropriate data technology, modern business intelligence (BI) would not be possible. BI relies on the ability to access, integrate, and transform data from disparate sources into a single, coherent view of the business. Data engineering lays the foundation for this by creating a robust, reliable and scalable data pipeline that can handle the volume, variety and velocity of data generated by modern businesses.
Data engineering enables companies to create a unified and accurate view of their data, essential for making informed decisions. By ensuring data quality and consistency, companies can trust their data and use it with confidence to gain insights and drive growth.
Additionally, data engineering is essential for managing and optimizing the performance of BI tools and applications. Without effective data engineering, BI solutions can suffer from slow query times, inconsistent data, and other issues that can limit their usefulness and impact.
In summary, data engineering is critical to modern business intelligence because it provides the infrastructure, processes and tools necessary to effectively collect, store, process and analyze data. Without data engineering, companies would struggle to get value from their data and would be at a disadvantage in today's data-driven business environment.
The importance of data engineering in building a strong analytics infrastructure
In the world of data analytics, a strong data engineering infrastructure is the foundation for success. Data engineering is the process of developing, building and maintaining the systems and processes that support the collection, storage, processing and analysis of data. Without a solid data engineering infrastructure, it will be difficult for companies to gain accurate and actionable insights from their data.
One of the key benefits of a strong data engineering infrastructure is the ability to process large amounts of data. With the explosion in data volumes in recent years, companies need to be able to process large amounts of data quickly and efficiently. Data engineering can help by creating pipelines that can process and move data at scale, allowing companies to keep up with growing data volumes.
Data engineering is also important to ensure data quality and consistency. Inaccurate or incomplete data can lead to incorrect insights and poor decisions. Data engineering processes such as data validation, cleansing, and transformation can help ensure data is accurate, complete, and consistent.
Another advantage of data engineering is the ability to integrate data from different sources. Companies often have data stored in different systems, formats and locations. Data engineering can help by creating systems and processes that integrate data from these sources and make it accessible for analysis.
Finally, data engineering is also essential for optimizing the performance of analytics tools and applications. Poorly designed data pipelines or inefficient processing can result in slow query times, which can be a significant bottleneck in the analysis process. A solid data engineering infrastructure can optimize query performance and enable companies to gain insights faster and more efficiently.
In summary, a strong data engineering infrastructure is essential to building a successful analytics program. By creating robust pipelines, ensuring data quality and consistency, integrating data from disparate sources, and optimizing performance, data engineering provides the foundation for extracting accurate and actionable insights from data.
How data engineering enables data-driven decision making
Those : questionpro.com
Data engineering is an important component for data-driven decision making in companies. Data-driven decision making means making decisions based on data analysis rather than intuition or guesswork. By using data engineering, companies can collect, process and analyze large amounts of data in a systematic and efficient manner, resulting in more informed and data-driven decisions.
Data engineering enables data-driven decision making by ensuring that data is accurate, complete and consistent. Data engineering processes such as data validation, cleansing and transformation help ensure the data is of high quality and can be used for decision making. This is important because inaccurate or incomplete data can lead to incorrect decisions.
Another way to make data-driven decisions is to integrate data from different sources. Companies often have data stored in different systems, formats and locations. Data engineering can help by creating systems and processes that integrate data from these sources and make it accessible for analysis. By integrating data from different sources, companies can gain a more comprehensive view of their business and make more informed decisions.
Data engineering can also help companies analyze data more efficiently. By creating data pipelines that can process and move data at scale, companies can gain insights faster and more efficiently. This is important because timely insights enable companies to respond to changing business conditions and make timely decisions.
Finally, data engineering can help companies develop predictive models and machine learning algorithms that can generate insights and predictions based on historical data. By using these models and algorithms, companies can make more accurate predictions about future events and make decisions accordingly.
In summary, data engineering is essential for data-driven decision making in companies. By ensuring data quality, integrating data from disparate sources, analyzing data more efficiently, and building predictive models, data engineering provides the foundation for extracting accurate and actionable insights from data.
The intersection of data engineering and business intelligence
Those: cio.com
Data engineering and business intelligence (BI) are two closely related disciplines that overlap in many ways. Data engineering is about developing, building and maintaining systems and processes that support the collection, storage, processing and analysis of data. BI, on the other hand, is about using data to gain insights and make informed business decisions. In this blog, we explore the intersection of data engineering and BI and how they work together to enable data-driven decision making in organizations.
One of the key overlaps between data engineering and BI is data integration. Data engineering processes can help companies integrate data from different sources and make it accessible for analysis in BI tools and applications. By integrating data from different sources, companies can gain a more comprehensive view of their business and make more informed decisions.
Data engineering and BI also overlap in the area of data modeling. Data modeling is the process of creating a data representation used in BI analysis. Data engineering processes can help companies create and maintain data models that are accurate, complete and consistent, enabling more effective BI analysis.
Another overlap between data engineering and BI is in the area of data processing. Data engineering processes can help companies process and move large amounts of data quickly and efficiently, which is important for generating timely insights in BI applications. By creating robust data pipelines, companies can ensure that data is processed in a timely and efficient manner, enabling more effective BI analysis.
Finally, data engineering and BI overlap in the area of data governance. Data governance involves establishing policies and procedures to manage data quality, security and compliance. Data engineering processes can help companies establish and maintain effective data governance practices, which are essential to ensuring that data is reliable, secure, and compliant with regulations.
In summary, the intersection of data engineering and business intelligence is critical to data-driven decision making in organizations. By integrating data from various sources, building accurate data models, processing data efficiently, and adopting effective data governance practices, companies can gain insights and make informed decisions that drive business success.
Data technology: The backbone of successful business intelligence
Those: intelligenthq.com
Data technology plays a crucial role in the success of modern business intelligence (BI) systems. As data volumes continue to grow exponentially, it becomes increasingly important for organizations to have a solid data foundation to ensure their BI systems are scalable and operate effectively. In this blog, we explore why data engineering is the backbone of successful BI and how it supports the delivery of valuable insights to decision makers.
One of the main reasons data engineering is so important to BI is that it involves developing, building, and maintaining the systems and processes that support the collection, storage, processing, and analysis of data. Without these systems and processes, it is impossible to effectively manage and analyze large amounts of data, which is a prerequisite for any successful BI system.
Data engineering also plays a crucial role in data integration, i.e. H. when combining data from different sources into a single, unified view. This is important because it allows decision makers to access a comprehensive view of their organization's data rather than working with data silos that are fragmented and incomplete. Data engineering processes can be used to ensure that data is integrated in a way that is accurate, complete and consistent, which is essential for producing reliable insights.
Another key role of data engineering for successful BI is data modeling. Data modeling is the process of creating a representation of the data that is used in analysis. By creating accurate and complete data models, companies can ensure that their BI systems provide reliable and relevant insights to decision makers. Data engineering processes can be used to ensure that data models are well designed and optimized for performance, which is essential for delivering timely insights.
Finally, data engineering is essential to ensure that data is stored and processed in a secure and compliant manner. Data security and compliance are critical for businesses across all industries, and failure to address these issues can result in costly fines and reputational damage. Data engineering processes can help organizations establish and maintain effective data governance practices that protect data from breaches and ensure compliance with relevant regulations.
In summary, data engineering is the backbone of successful business intelligence systems. By providing systems and processes to support data collection, storage, processing and analysis, data engineering enables companies to generate reliable insights that support data-driven decision making. Without a solid data engineering foundation, BI systems will struggle to scale and provide valuable insights to decision makers.
Building a future-proof business intelligence strategy with data engineering
Those: smartdatacollective.com
In today's rapidly evolving business landscape, it is becoming increasingly important for companies to develop future-proof business intelligence (BI) strategies that can adapt and evolve over time. Data engineering plays a critical role in this process as it provides the systems and processes that support the collection, storage, processing and analysis of data. In this blog, we explore how companies can use data engineering to build a future-proof BI strategy that delivers valuable insights and supports data-driven decisions.
The first step in building a future-proof BI strategy is to create a solid data foundation. This requires developing and building the systems and processes to support data collection, storage and processing. By investing in a robust data engineering infrastructure, companies can ensure that their data is managed effectively and efficiently and that their BI systems can scale and evolve over time.
One of the key benefits of using data engineering to build a future-proof BI strategy is that it allows companies to integrate data from different sources. This is important because it allows decision-makers to access a comprehensive view of their organization's data, rather than having to work with fragmented and incomplete data silos. Data engineering processes can be used to ensure that data is integrated in a way that is accurate, complete and consistent, which is essential for producing reliable insights.
Another important aspect of building a future-proof BI strategy with data engineering is data modeling. Data modeling is the process of creating a representation of the data that is used for analysis. By creating accurate and complete data models, companies can ensure that their BI systems provide relevant and reliable insights to decision makers. Data engineering processes can be used to ensure that data models are well designed and optimized for performance, which is essential for delivering timely insights.
Data engineering is also crucial to ensure that data is stored and processed in a secure and compliant manner. This is particularly important in today's regulatory environment, where data security and compliance are top priorities for companies across all industries. By adopting effective data governance practices, companies can protect their data from breaches and ensure compliance with relevant regulations.
In summary, data engineering is a critical component in building a future-proof BI strategy. By providing the systems and processes that support data collection, storage, processing and analysis, data engineering enables companies to generate valuable insights that support data-driven decision making. By investing in a robust data engineering infrastructure, companies can ensure that their BI systems can adapt and evolve over time, keeping them ahead of today's rapidly changing business landscape.
The key components of a robust data engineering strategy for business intelligence
Those: firsteigen.com
A robust data engineering strategy is a critical component of any successful business intelligence (BI) system. Data engineering involves the development and construction of the systems and processes that support data collection, storage, processing and analysis. This blog introduces the key components of a solid data engineering strategy for BI and how they enable organizations to gain valuable insights that support data-driven decision making.
The first component of a robust data engineering strategy is data integration. Data integration combines data from different sources into a single, unified view. This type of data integration allows companies to avoid the pitfalls of working with fragmented and incomplete data silos and gives decision makers a comprehensive view of their organization's data. Data engineering processes can be used to ensure that data is integrated in a way that is accurate, complete and consistent, which is essential for producing reliable insights.
The second component of a solid data engineering strategy is data modeling. Data modeling is the process of creating a representation of the data that is used in analysis. By creating accurate and complete data models, companies can ensure that their BI systems provide relevant and reliable insights to decision makers. Data engineering processes can be used to ensure that data models are well designed and optimized for performance, which is essential for delivering timely insights.
The third component of a solid data engineering strategy is data processing. Data processing involves converting raw data into a format suitable for analysis. This can include tasks such as cleaning data, enriching data with additional information, and aggregating data. By using data engineering processes to optimize data processing, companies can ensure that their BI systems deliver accurate and relevant insights.
The fourth and final component of a solid data engineering strategy is data governance. Data governance involves establishing policies and procedures for data management and compliance with relevant regulations. This is particularly important in today's regulatory environment, where data security and compliance are top priorities for companies across all industries. By adopting effective data governance practices, companies can protect their data from breaches and ensure compliance with relevant regulations.
In summary, a solid data engineering strategy is a critical component of any successful BI system. By focusing on data integration, data modeling, data processing and data governance, companies can ensure their BI systems provide valuable insights that support data-driven decision making. Investing in a solid data engineering foundation can help companies stay ahead in today's rapidly changing business landscape.